Rise of the Reasoning Models
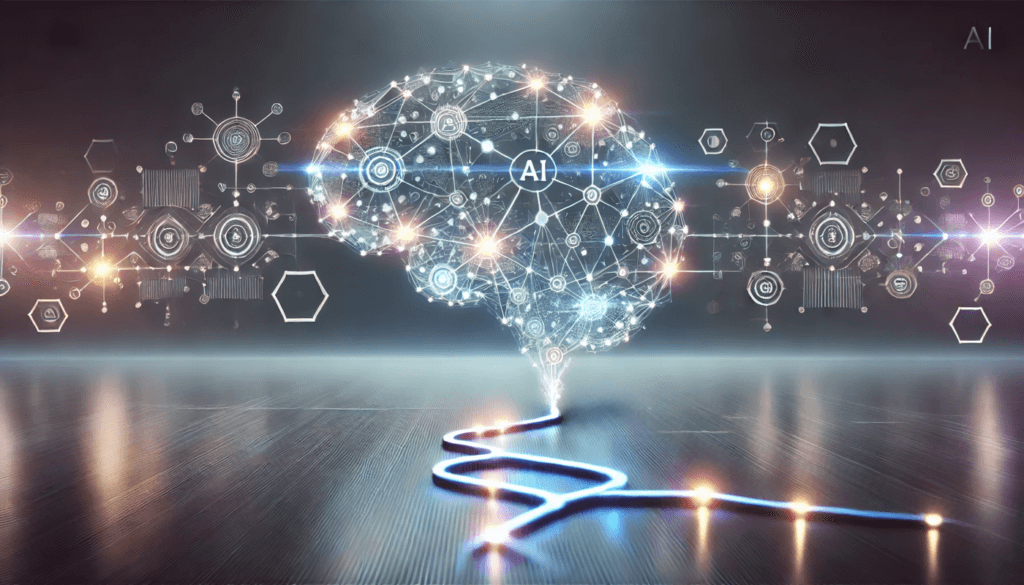
Last week, I sat on a panel at the Maryland Technology Council’s Technology Transformation Conference to discuss Data Governance in the Age of AI alongside an incredible group of experts. During the Q&A, someone asked about DeepSeek and how it changes how we think about data usage—a question that speaks to a fundamental shift happening in AI.
When I give talks on AI, I often compare foundation models—AI models trained on vast datasets—to a high school or college graduate entering the workforce. These models are loaded with general knowledge, and just like a college graduate or a master’s degree holder, they may be specialized for particular industries.
If this analogy holds, models like ChatGPT and Claude are strong generalists, but what makes a company special is its secret sauce—the unique knowledge, processes, and experience that businesses invest heavily in teaching their employees. That’s why large proprietary datasets have been key to training AI, ensuring models understand an organization’s way of doing things.
DeepSeek changes this approach. Unlike traditional AI models trained on massive datasets, DeepSeek was built on a much smaller dataset—partly by distilling knowledge from other AI models (essentially asking OpenAI and others questions). Lacking billions of training examples, it had to adapt—which led to a breakthrough in reasoning. Instead of relying solely on preloaded knowledge, DeepSeek used reinforcement learning—a process of quizzing itself, reasoning through problems, and improving iteratively. The result? It became smarter without needing all the data upfront.
If we go back to that college graduate analogy, we’ve all worked with that one person who gets it. Someone who figures things out quickly, even if they don’t have the same background knowledge as others. That’s what’s happening with AI right now.
Over the last few weeks, every major AI company seems to be launching “reasoning models”—possibly following DeepSeek’s blueprint. These models use a process called Chain of Thought (COT), which allows them to analyze problems step by step, effectively “showing their work” as they reason through complex tasks. Think of it like a math teacher asking students to show their work—except now, AI can do the same, giving transparency into its decision-making process.
Don’t get me wrong—data is still insanely valuable. Now, the question is: Can a highly capable reasoning model using Chain of Thought deliver answers as effectively as a model pre-trained on billions of data points?
My guess? Yes.
This changes how companies may train AI models in the future. Instead of building massive proprietary datasets, businesses may be able to pull pre-built reasoning models off the shelf—just like hiring the best intern—and put them to work with far less effort.